Nightshade and A.I. Image Poisoning
While copyright owners with resources fight A.I. developers in court and governments scramble to regulate the transformative technology, a university team’s release of Nightshade offers visual creatives a subversive tool to poison any image an A.I. model tries to digest.
Late last year, University of Chicago Professor Ben Zhao and his team at the SAND Lab announced Nightshade, a program that can make any image unrecognizable to even the biggest A.I. models through a process known as data poisoning.
On January 18, it was released:
Nightshade has received 250,000 downloads in the first five days of its release.
Some see data poisoning as a human rebellion against the technology threatening their livelihood. Others poison their content to protect their brand against the risk of poor-quality reproductions floating around. But for small and independent creators, data poisoning is a practical method to protect their work and professional identity from being repurposed for free.
However some proponents of A.I. technology acceleration claim that data poisoning is pointless because datasets used to train foundation models are too massive to be ruined by small samples of corrupted data. Critics of data poisoning also predict that A.I. developers will find ways to revert poisoned images back to their true form.
Until there are laws and/or legal precedents to protect artists from an invisible machine indiscriminately scraping their content to learn from, data poisoning may be artists’ best chance to keep control of their content in the meantime.
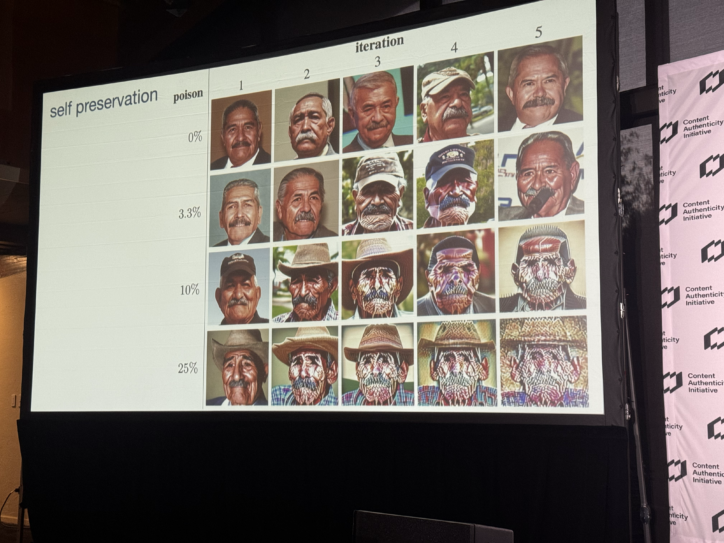
Why Would Artists Resort to Poison?
The advent of A.I. has complicated the landscape for creators over the past year in multiple ways. Not only do artists have to worry about autonomous A.I. agents eliminating their jobs, but even the work they do publish online is at risk of being commoditized by an A.I. model capable of spitting out a million variations of anything it sees. Publishing work online assumes the risk of that work being copied, and then used to make the A.I. model better at copying and reproducing other works.
Because generative A.I. is such a new phenomenon, we don’t have robust regulations and mechanisms in place to protect content creators or empower them to seek relief when an A.I. model helps to reproduce copyrighted work without permission. Meanwhile, lawyers for OpenAI recently acknowledged that A.I. developers are incentivized to scrape web content in order to keep their models relevant for modern uses. Developers argue in pursuit of unfettered access to the world’s content that training A.I. models on copyrighted works should be considered a fair use.
Content owners of with the means are fighting back against A.I. companies scraping all kinds of content. The stock photo and image licensing platform, Getty Images, claimed in a lawsuit last year that Stability AI trained its image generator, Stable Diffusion, on unlicensed versions of an estimated 20 million images in Getty’s database. Part of Getty’s supporting evidence includes images generated by Stable Diffusion that clearly show poor reproductions of the Getty logo.
A group of artists also sued Stability AI, Midjourney, and the artist platform Deviantart last year claiming that the A.I. image generators developed by each defendant were trained with copyrighted images scraped off the internet.
In the world of writing and publishing, multiple authors and the New York Times launched similar lawsuits claiming that A.I. models are digesting their writing and repurposing it in user-prompted output without compensation to the creator.
On the whole, the decisions that come down in these lawsuits will have wide effects across the business of content production. However, it could take years for those arguments to play out. The U.S. Copyright Office is also working to develop proposals for rules changes in the era of generative artificial intelligence. However, the outcome of those processes are of no help to creators here and now.
That’s where a tool like Nightshade comes in.
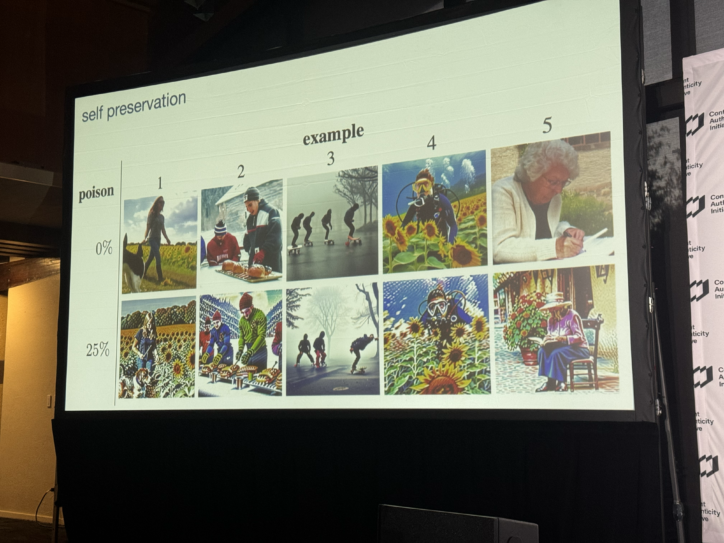
How does Nightshade work?
Nightshade is data poisoning specifically for images. Its creators say that it’s effective at fooling A.I. models and impossible to bypass.
The idea behind data poisoning is similar to mixing up the labels in a filing cabinet. If the data isn’t labeled correctly or it’s in the wrong location, it can be useless or even detrimental to the larger system. If you’re preparing for a presentation about dogs using a folder titled “Dogs” that contains information exclusively about cats, you might get laughed off stage. Similarly, you’d be annoyed if you asked your A.I. model for a picture of a flower and it generated an image of a rock. Little did you know that the A.I. just went to the folder for “flowers,” and instead found pictures of rocks put there by Nightshade.
Nightshade works by manipulating the actual image data that the A.I. model reads. The technology changes pixels’ color coding so subtly that it’s undetectable to the human eye, but an A.I. model is tricked into interpreting the image as something else. So a human annotator would see the image and label it for what it is, which is different from what the A.I. would perceive in the image data. A poisoned picture of a dog may look like a cat to an A.I. model, or a hat may look like a cake. UChicago researchers proved that less than 25 percent of a model’s dataset needs to be corrupted to make the output images unusable. Beyond 25 percent, output images for dogs start to clearly look like cats.
The practical effects of the technology on real-world LLMs are hard to predict. Nightshade’s founders say that the tool is effective for “prompt-specific” poisoning, in other words: it works better on more specific topics. Think about how many pictures of dogs are on the internet, so many that A.I. models may know more about dogs than humans. It would be difficult to poison enough of the images in that broad dataset to force an A.I. model to reassociate the word “dog” with an image of a cat. But imagine you’re a digital comic book illustrator who’s developed a particularly edgy style, or a unique character that others may want to try to copy. Since you own all of the images associated with your name, you can put them all through Nightshade before publishing them online. Now all (or a majority) of the reference images available to an A.I. model are corrupted. So if your image goes viral and somebody else tries to benefit by generating and sharing knockoffs, the A.I. image generator can only rely on the poisoned images to build the output. Once that data is corrupted past a certain point, it’s much harder for an A.I. model to regain the correct understanding.
So while Nightshade’s effectiveness is limited to very specific topics, it does offer a measure of protection for creators who have spent the time and energy to develop unique content.
But could Nightshade actually damage the usefulness of A.I. models? Probably not, except where there are serious efforts to poison a lot of content in highly concentrated conceptual spaces. LLMs have wealths of reference material to choose from all the training data that already exists. Some speculate that A.I. developers already have or will have technology that can weed out poisoned images so they don’t affect the model’s output. It’s missing the point, though, to say that Nightshade may be ineffective because an A.I. model could be trained not to learn from a poisoned image. Rather than “fighting back” against A.I., many artists simply want fair compensation for their work. Regardless if Nightshade doesn’t stop the rise of A.I., it puts a price on web scraping for developers and forces them to think critically about the data they use for training.
Blocking A.I. Models to Learn Artists’ Style with Glaze
Zhao’s’ team also created Glaze, a tool to prevent A.I. models from learning an artist’s signature “style.” This is done similarly to Nightshade, where pixels are manipulated for the ML algorithms but don’t impact the appearance to humans. Since it was released in April of 2023, it’s seen 2.2 million downloads.
From Nightshade to a New Day for A.I.
Prof. Zhao acknowledges that there will likely come a time when technology or law makes Nightshade obsolete. Somebody could develop code that reverses Nightshade’s effects. Preferably and more likely, laws and copyright rules will eventually catch up with the technology.
The damage has already been done to artists whose work is available online. But until we have mature regulations in place to balance the rights of content owners with the economic and cultural appeal of A.I., Nightshade could help new artists and those to come have the confidence and peace of mind to publish online.
If nothing else, Nightshade is contributing to a discussion about how A.I developers source their training data, what say the owners of that data should have, and how we as a culture value the creators of the content we consume versus the convenience of an A.I. already trained on all the content we know and love.