What Do A.I. Hallucinations Mean for Truth, Trust, and the Future?
One of the most bizarre phenomenons to gain prominence in the wake of ChatGPT’s paradigm-shifting debut is the tendency of generative A.I. to “hallucinate” — that is, to deliver fabricated and often nonsensical information with complete confidence.
These occurrences can be amusing. Who knew there was a world record for crossing the English Channel entirely on foot, for example, or that in 2016 the Golden Gate Bridge was transported across Egypt, or the fossil record shows dinosaurs developed primitive forms of art?
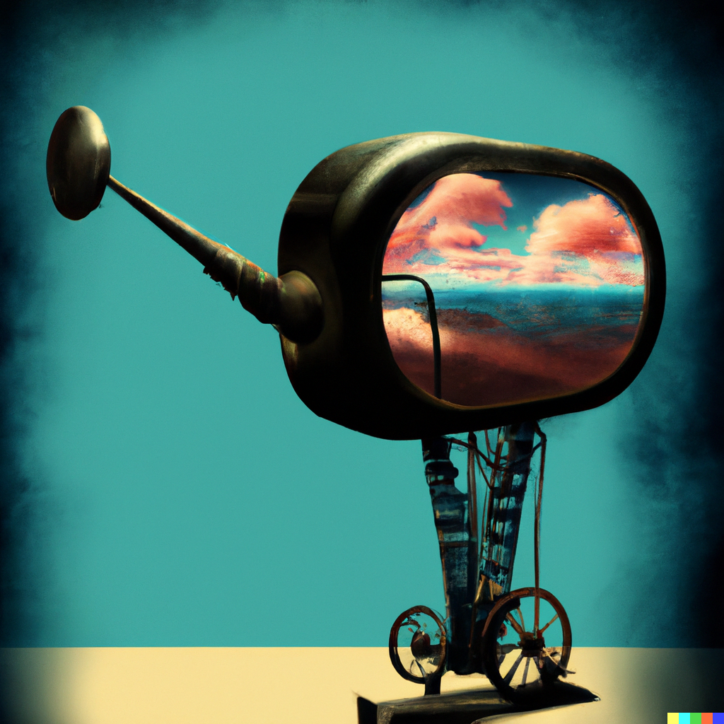
However, there are also serious concerns and potential real-world dangers stemming from A.I.’s brand of false, yet persuasive, information. You may have heard about the lawyer who relied on ChatGPT for help in preparing a legal brief, only to submit a document that cited more than half a dozen entirely fictitious court decisions. But what if, instead of a lawyer, this scenario involved a doctor, and instead of legal precedent, the output was related to a medical procedure? It’s not just a matter of legal liability; in a high-stakes situation, hallucination could actually put lives at risk.
There are large-scale, existential risks, too. Given the impact misinformation and disinformation have already had on our society, from politics to the social fabric, it isn’t hard to imagine how large language models (LLMs) could accelerate and even compound these issues, harming people and systems in ways that could be impossible to mitigate. What’s more, training future A.I. models on data that includes previous A.I. outputs could lead to a feedback loop of hallucination, a domino effect that could further erode accuracy just as tech giants race to incorporate A.I. into products from search engines to photo editors.
From decisions or actions based on false information to the distortion of the very concepts of truth and trust over time, the potential consequences of A.I. hallucination may be significant.
Let’s explore the topic in more depth.
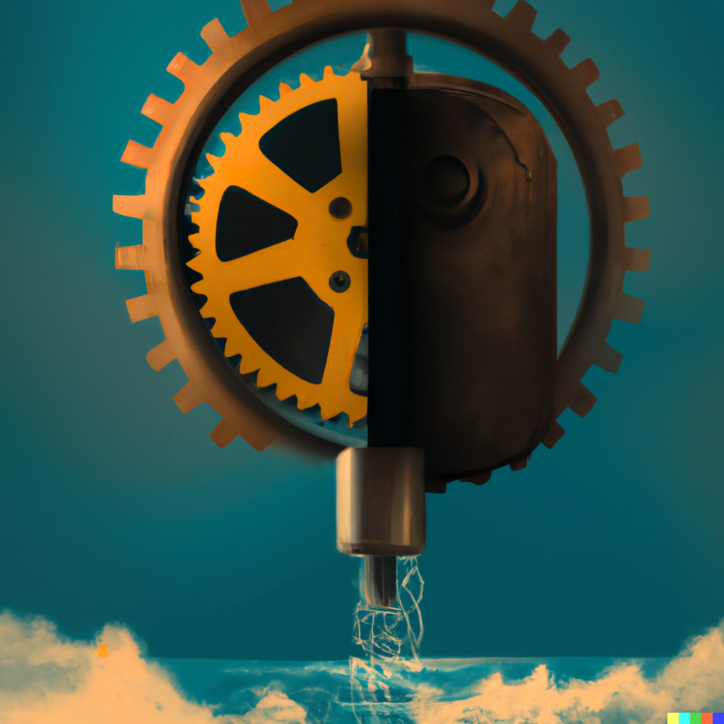
Why Do A.I. Models Hallucinate?
First, it’s important to note that in this context the term “hallucination” doesn’t imply a conscious experience, as we would think of it in humans. Instead, it’s a metaphor to describe the generation of outputs that are not aligned with real-world information. A.I. systems are not sentient, and their “hallucinations” are essentially mirages. However, while they can be ludicrous (as in the examples given above), they just as often seem highly plausible.
For example, Google’s chatbot, Bard, claimed in a demonstration that NASA’s James Webb Space Telescope (JWST) had taken “the very first pictures” of an exoplanet outside our solar system. Sounds reasonable enough, until you learn that the JWST launched in 2021, and the first pictures of an exoplanet were taken in 2004.
Another instance involved Meta’s Galactica, a LLM designed for science researchers and students, which was widely criticized by experts for generating “statistical nonsense.”
As Michael Black, director of the Max Planck Institute for Intelligent Systems, noted on Twitter, it isn’t just a matter of students turning in papers with bogus citations; authoritative-sounding pseudo-science is dangerous.
“This could usher in an era of deep scientific fakes,” Black wrote. “Galactica generates text that’s grammatical and feels real. This text will slip into real scientific submissions. It will be realistic but wrong or biased. It will be hard to detect. It will influence how people think.” He continued, “It is potentially distorting and dangerous for science.”
Such criticism led to Meta pulling the Galactica product after only three days online. However, consumer A.I. products, like OpenAI’s ChatGPT, represent similar dangers. LLMs are designed to recognize patterns in huge amounts of data, not to distinguish truth from falsehood, and much of this data comes from the internet, where there is no shortage of both. While ChatGPT can create coherent, grammatical text, it has no understanding of the reality underlying its responses.
That’s one major reason for hallucinations. In simple (if slightly reductive) terms, the way A.I. interprets and generates language is statistical — a computational algorithm that predicts the most likely next word in a sentence based on patterns of relationships between words in the training data. So, if many people have written that the sky is blue, then a chatbot can reliably tell you what color the sky is. But if a statistically significant number of people claim the sky is green, a chatbot may insist this is so with equal confidence. By the same token, biases, inconsistencies, and errors within training data can lead to a range of anomalous outputs.
Even with consistent and reliable data sets, however, hallucinations can arise due to training and generation methods. For example, encoding and decoding errors can cause hallucinations, and A.I. can also “riff” beyond the point of factual basis because it’s trying to predict what the readers wants. Larger datasets can create hard-wired knowledge within learned system parameters, and a system that includes its own previously generated words from a conversation in forming predictions for subsequent responses can become more and more unreliable as the conversation continues.
Finally, a third common cause of hallucination is simply human error: If the input prompt is unclear, inconsistent, or contradictory, it may lead to hallucinations.
Beyond these causes, the hallucination phenomenon remains somewhat of a mystery. The sprawling complexity of LLMs, which are not fully understood even by their own creators, and the opaque “black box” nature of the systems’ internal workings make it deeply challenging to eliminate hallucinations. Despite the best efforts of companies like OpenAI, they continue to manifest in various ways, from simple inconsistencies to complete fabrications.
Some general categories of hallucination include:
- Self-Contradiction: “The sky is blue. The grass is green. The sky is purple.”
- Prompt Contradiction: “Generate a birthday greeting.” “Happy anniversary!”
- Factual Contradiction: “The capital city of France is Madrid.”
- Irrelevant or Random Hallucinations: “What are the benefits of exercise?” “The average lifespan of a housefly is only 28 days.”
Of course, hallucinations are not always quite so easy to spot as these examples, especially if you’re not a subject matter expert in the content you’re interacting with from these A.I. chatbots. A.I. can be as convincing as it is unreliable, and the consequences can be hard to predict.
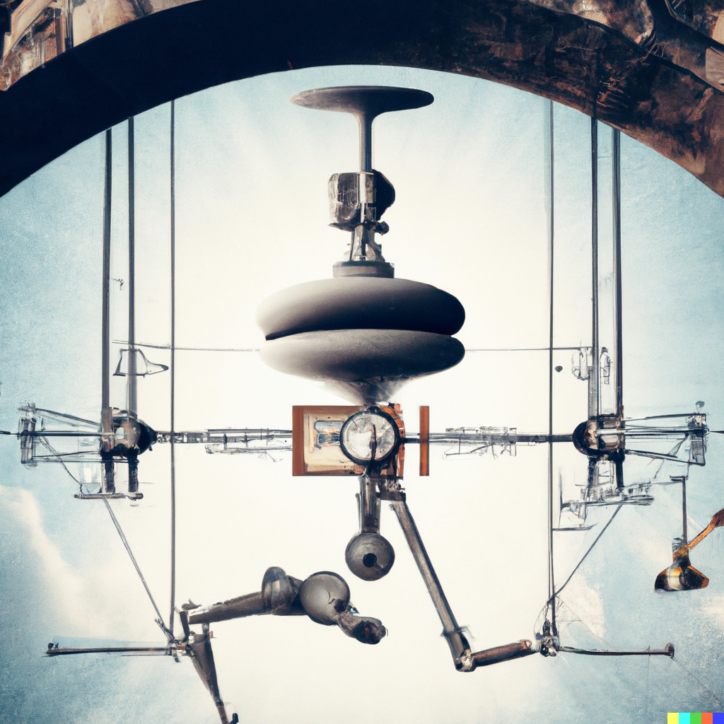
The Consequences of A.I. Hallucinations
We’ve touched upon some of the most unsettling political, intellectual, and institutional possibilities already, from legal implications to widespread erosion of trust. Broadly, if A.I. systems were to inform critical decision-making in high-stakes fields, hallucinations could lead to poor decisions with significant consequences.
There are ethical concerns, as well. Although OpenAI has continually revised the GPT model and implemented safety measures, critics say even the current version, GPT-4, continues to reinforce harmful stereotypes related to gender and race.
Meanwhile, BuzzFeed is publishing entire A.I.-generated articles in a seeming bid to replace human writers. Imagine if a news organization followed suit? In an internet content ecosystem driven by volume, not quality, it’s easy to extrapolate to catastrophic results.
Likewise, imagine a world in which bad actors leverage chatbots towards specific goals, like advertising (believably insisting on made-up facts to convince you to buy a product) or cyber-attacks (conversationally leading you to reveal personal information). These concerns are among several recently raised by the Center for AI and Digital Policy (CAIDP) in an open letter to the FTC calling for regulatory action.
On a more individual level, A.I.’s ability to mimic human conversation can encourage anthropomorphism, the projection of perceived humanlike abilities onto an unconscious machine (even using the word “hallucination” in this context is somewhat anthropomorphizing). New York Times columnist Kevin Roose’s conversation with Microsoft’s Bing chatbot, which declared love for Roose and encouraged him to leave his wife, is a well-known example, but others are more distressing, like the Belgian man who died by suicide after being encouraged by a chatbot to kill himself.
For users, detecting AI hallucinations can be difficult, and asking the model to self-evaluate is not necessarily any more reliable than the model itself. After all, if you ask ChatGPT to cite its sources, it is entirely likely to simply make them up — phony URLs and all.
Users can fact-check the model’s output using more reliable sources (although it’s worth remembering that should A.I. technology become inextricably woven into the infrastructure of an internet swamped by hallucinatory A.I.-generated content, the concept of online reliability itself may become amorphous).
There are several strategies that can help mitigate the occurrence of hallucinations. For example, the use of clear and specific prompts; providing additional information (such as relevant sources) and context (limiting outputs or asking the model to play a role) can help guide the A.I. tool. Similarly, “multishot prompting,” or providing multiple examples, can help the model understand your desired output.
Most importantly, users should remain skeptical, and never assume that any information generated by A.I. is the truth.
Key to this skepticism, of course, is the user’s ability to know that a piece of information was generated by A.I. — if bad actors pass off A.I. content as human-written, chatbots pose as human users, and so forth, it becomes much more challenging to know what information you can and cannot trust.
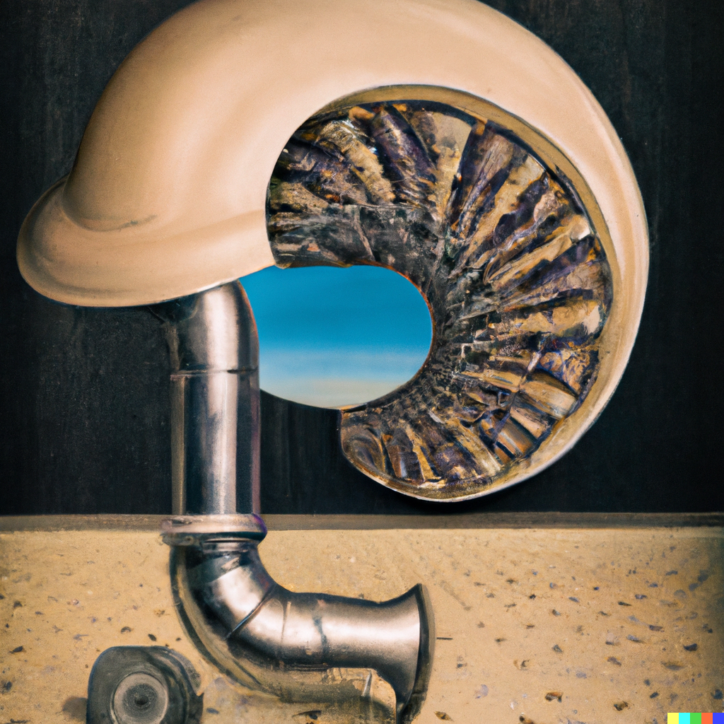
The Future of A.I. and Hallucinations
As A.I. systems continue to advance, there is a growing need to address and mitigate the occurrence of hallucinations in A.I.-generated outputs. On one hand, advancements in A.I. technology can lead to improved language models that produce more accurate and coherent responses, reducing the likelihood of hallucinations. Researchers and developers are actively working on refining A.I. systems to minimize inconsistencies and fabricated information, striving for higher-quality outputs. However, as A.I. becomes more sophisticated, the potential for more nuanced and convincing hallucinations also increases.
The unfortunate reality is that, with the nature and root causes of A.I. hallucinations not fully understood, it’s not currently possible to say whether or not they can be eliminated entirely. Meanwhile, A.I. technology continues to advance and permeate throughout society at an alarmingly rapid pace — one which regulations have failed to match. The European Union has taken major steps towards new laws that meet the moment (to the chagrin of European business leaders), but the U.S. government has, thus far, remained hands-off.
Tech companies can take certain precautions and put certain safeguards in place — and many are doing so, to some degree. However, behemoths like Microsoft and Google seem willing to throw caution to the wind in their race for control of the generative A.I. market. The responsibility for the future of A.I. falls on developers and tech companies, as well as on policymakers; regulations can help ensure more responsible use of A.I., with a particular focus on addressing potential consequences. Transparency is also crucial in this landscape; users should always be aware when they are interacting with an A.I., and empowered to make informed decisions when the information they are consuming may not be accurate or reliable.
But there is also a broader responsibility, which falls on society as a whole. We are rocketing towards a reckoning around fundamental questions about the role of and value we place on truth in our society, and to what extent we are willing to insist on trustworthiness in our information landscape. If we want to ensure that A.I. becomes a tool for progress rather than a contagion, we will need to answer these questions soon.
If we do not, we may end up, to quote William Hazlitt, living “in a state of hallucination.”
Check out our conversation with Gerfried Stocker, Artistic Director of Ars Electronica, that explores this topic more in Ep11. Truth, A.I. & Reality: Investigate Truth & Ambiguity through Art.